I agree with you it’s not the most elegant solution but it’s the solution that requires the least amount of effort. To do this properly and merge upstream we’d need to extend the landing target protocol specification and implement support for this new implementation in both PX4 and Ardupilot – these are the “rules” of contributing to mavlink and this process can be quite time consuming.
You might be interested in this recent PR
PX4:main
← JonasPerolini:pr-land-target-refactor
opened 01:38PM - 22 Nov 22 UTC
**Goal**: Extend the current landing target estimator to:
- be more precise:… properly include uncertainties in the filter and allow to use coupled dynamics.
- be more robust: use more independent sensors
- have separated dynamics for moving targets.
- estimate the full pose of the target
**Use case**
- Be able to land combining both vision (or uwb , irlock) and GPS under large measurement uncertainties. The GPS can come from a GPS on the target or simply using the mission position.
- Land on moving targets equipped with a GPS.
**Main changes:**
1. `LTEST_AID_MASK`: chose which measurements to sequentially fuse
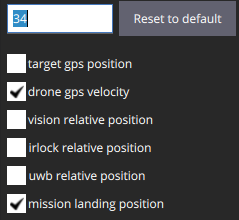
3. `LTEST_MODE`: chose between coupled and decoupled dynamics (trade off between speed and accuracy. Since we perform the fusion in vehicle-carried NED frame, the x,y,z directions are coupled)
4. `LTEST_MODEL`: chose the KF model between static and moving targets. (see _documentation/KF_models.pdf_)
5. `LTEST_BTOUT`: timeout after which the landing target is considered lost without any new position measurements. Before the filter times out, the prediction (obtained with the mathematical models described below) is used to estimate the target position.
6. Estimate the full target pose (x,y,z,theta).
7. Extend the state to estimate the bias between the GPS unit on the drone and the GPS on the target (or the mission land pose).
8. Properly log observations and innovations (using _`estimator_aid_source_3d.msg`_)
9. Deal with time sync issues (useful for vision measurements which require image processing). To compute the innovation, the filter state is moved back in time to be synced to the measurement time of validity
10. Complete messages to include uncertainties
**Mathematical models**
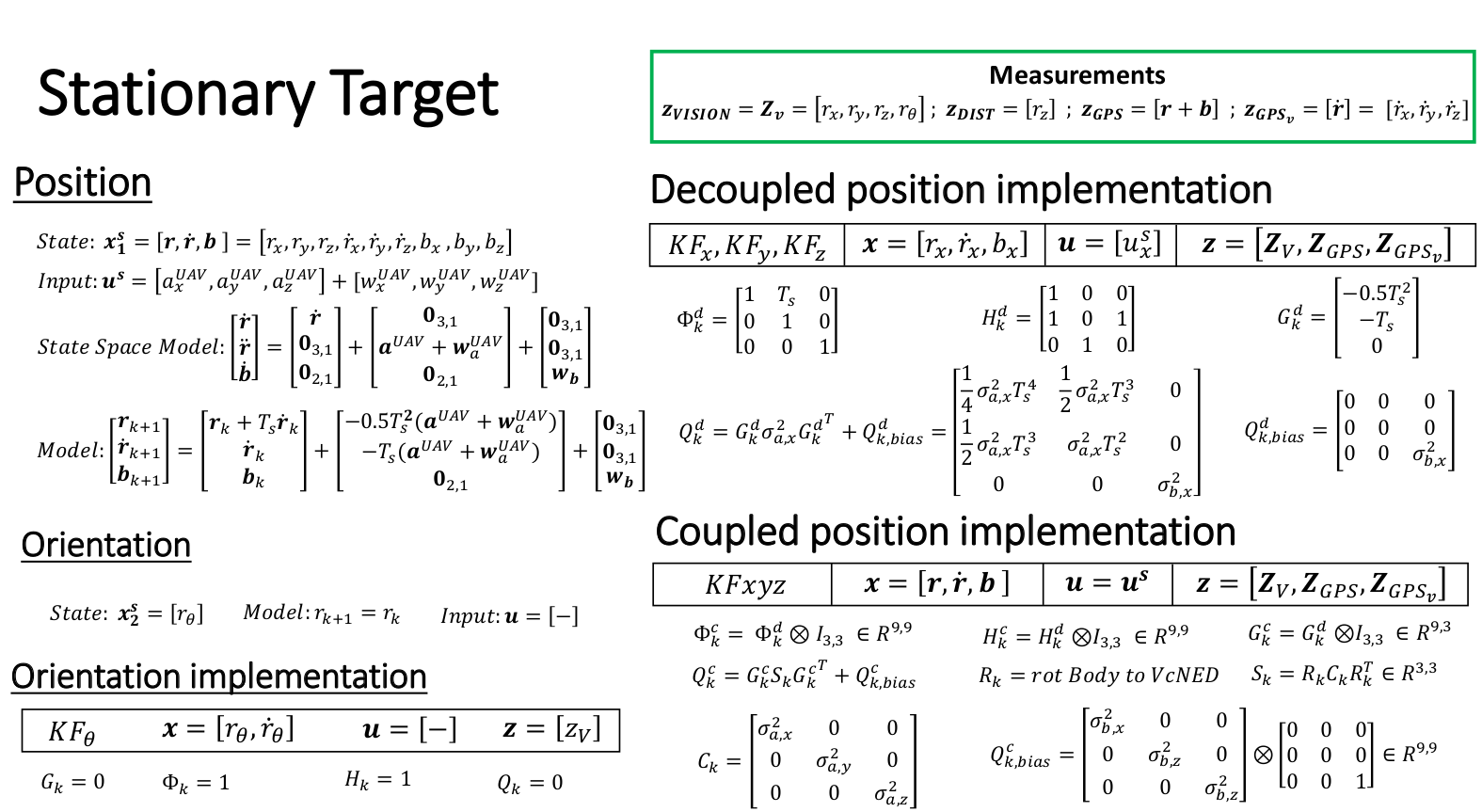
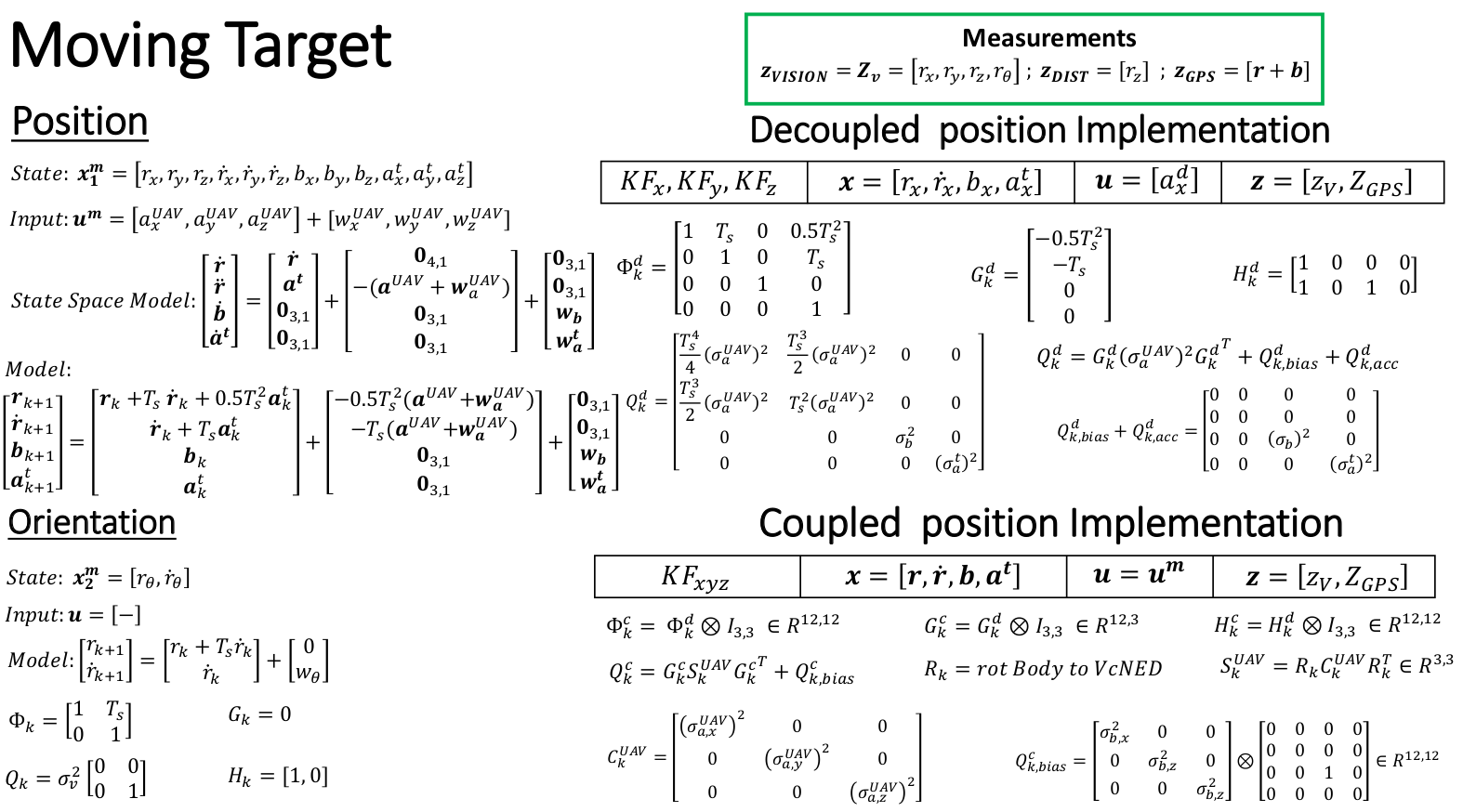
- Note: The estimator is still compatible with the previous implementation. However there are some changes:
1. Compensate for gravity in the acceleration
2. Publish the target using the prediction step if no measurement is available (until timeout is reached)
3. Use proper timestamp
**TODO**
- Reduce allocated stack (for now 6000)
- Stop the target estimator module once disarmed
- Complete the landing target MAVLINK message to include measurement covariance matrix
- Diagonalize the measurement matrix to properly use sequential update
- Get drone's acceleration uncertainty
- Orientation (the precision landing algorithm doesn't take the orientation into consideration yet).Define initial values && Complete MAVLINK landing target message to include theta
- Add (x,y,z) filters when no bias is required.
**Structure overview:**
- Target estimator class:
--- _target_estimator_: Defines the decoupled target estimator class. Contains the virtual funct. that each KF model will inherit
--- _target_estimator_coupled_: same as _target_estimator_ but for coupled dynamics
- Mathematical model of the filters derived using _SymForce_ (see _documentation/Python derivation_):
--- _KalmanFilter_
--- _KFxyzCoupledMoving_
--- _KFxyzDecoupledMoving_
--- _KFxyzCoupledStatic_
--- _KFxyzDecoupledStatic_
- Main module: _LandingTargetEstimator_
1. Initializes the KF instances e.g. for a static target and decoupled dynamics: init 3 filters (for x,y,z directions) with the same KF model (_KFxyzDecoupledStatic_).
2. Sub to relevant topics to get the available measurements
3. Handles the Kalman filter sensor fusion (init, prediction and update step)
5. Publishes the landing target and innovations
**Preliminary results**
- Flight test with: stationary target, coupled dynamics fusing GPS velocity and external vision. https://logs.px4.io/plot_app?log=ede59db8-18ed-4ce1-85e6-863c9b2d61a3 The estimator is init at `(0.8, 2.8, 7)`. The drone then corrects horizontally before descending above target and land.
https://user-images.githubusercontent.com/74473718/203308784-c8bcb0a0-9b42-4cc0-bfd2-f0f870c0cd00.mp4
The filter smooth-ens the target estimation (see top plot). It also allows to detect outliers in the vision measurements (most likely due to time sync issues) and still use the prediction model to properly estimate the target position.
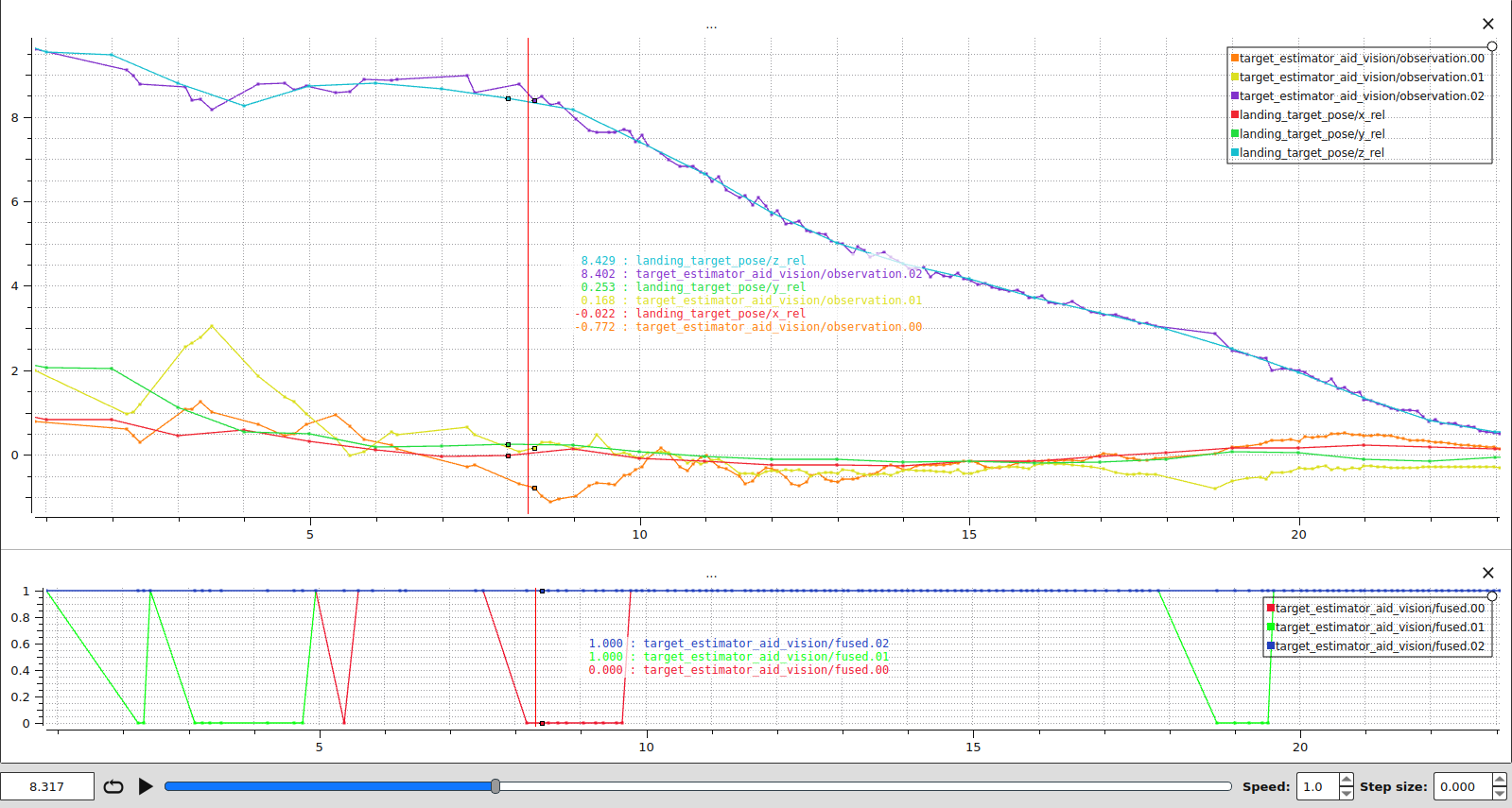
Once again, the velocity estimation is much smoother than the observation.
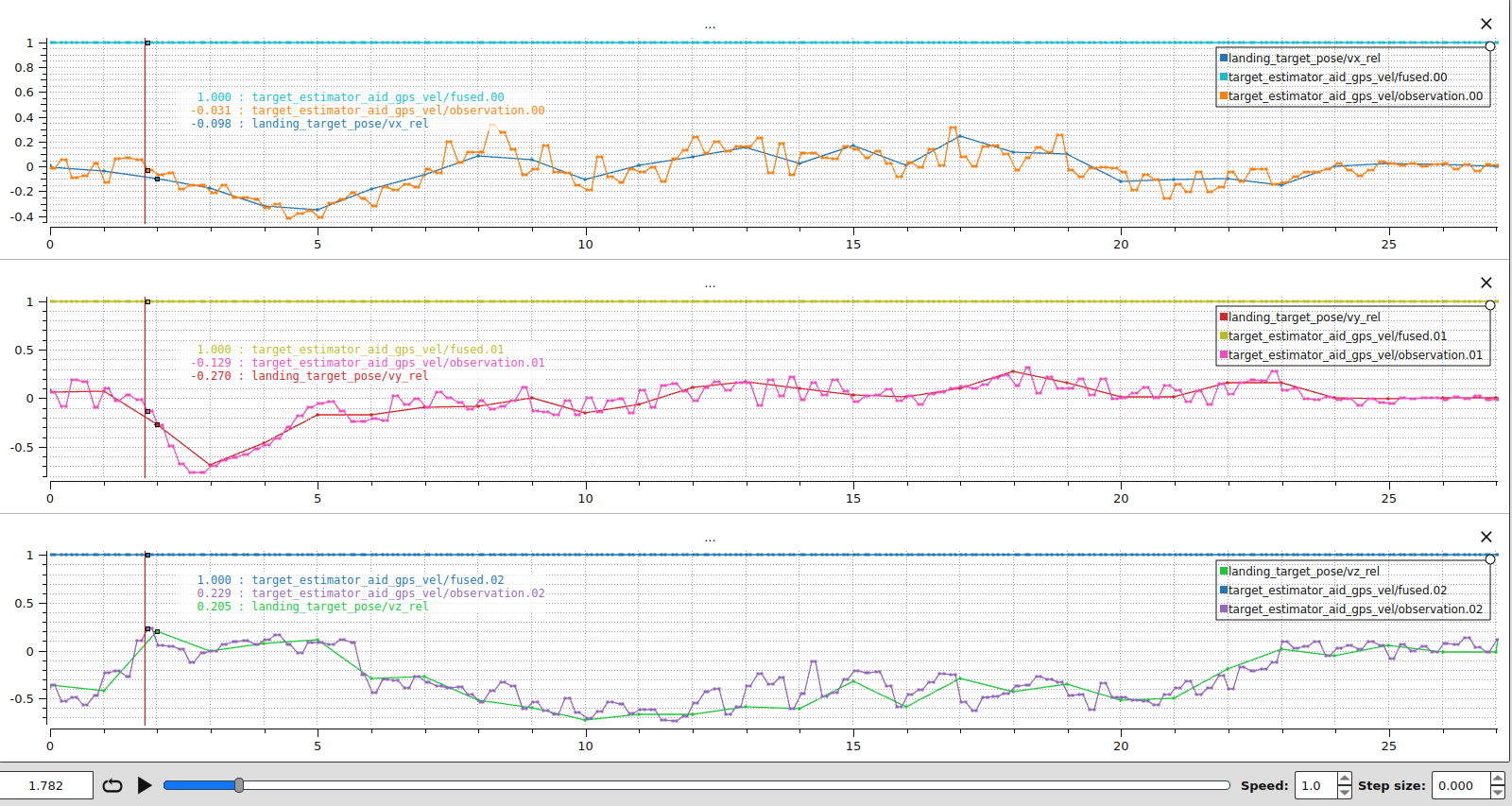
The drone correctly navigated towards the target. Since the drone landed on the target (see video above) we can conclude that the target was correctly estimated.
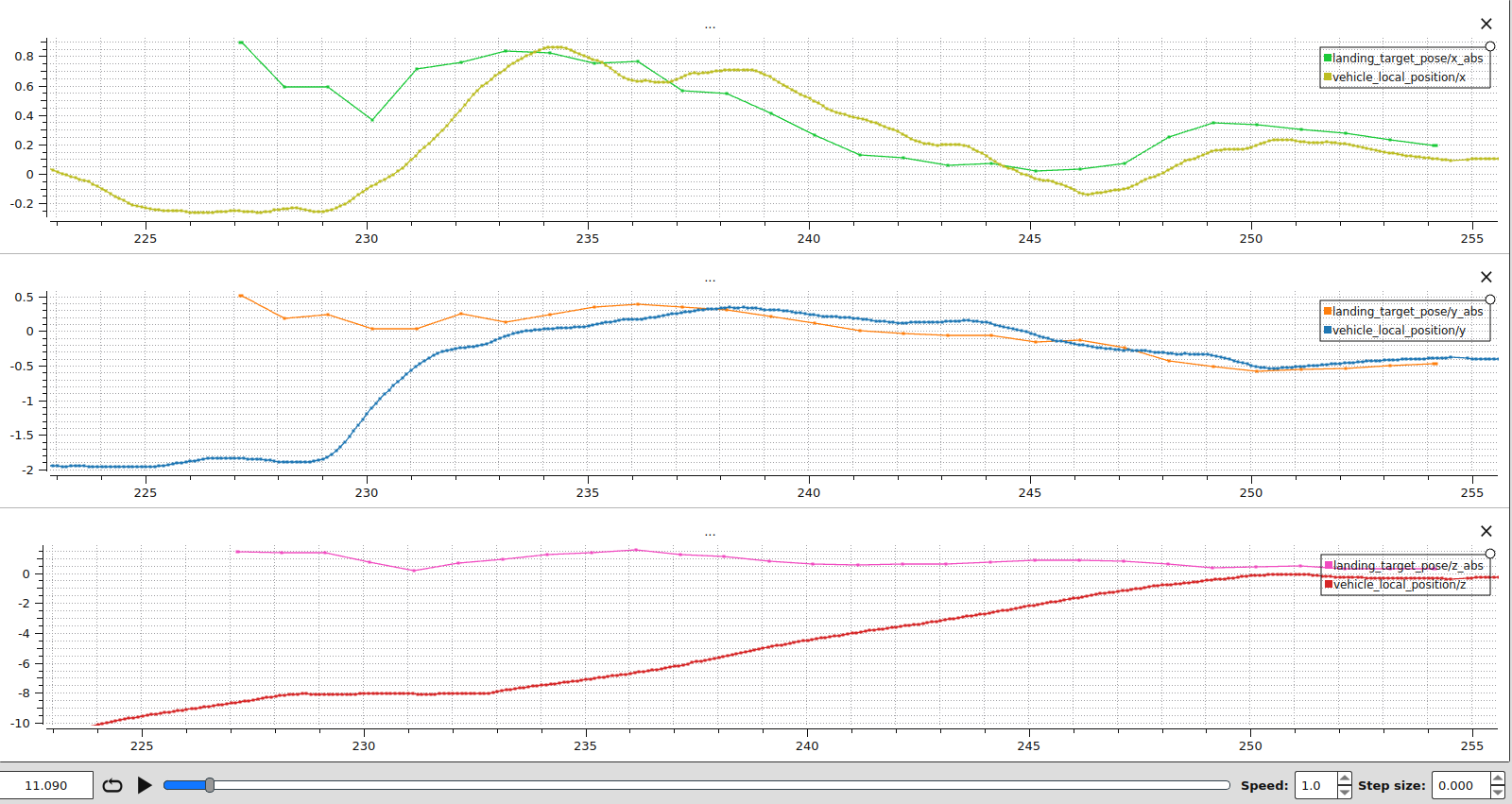
CPU usage: the sensor fusion (matrix multiplication) starts once the next waypoint is of type land. As the fusion starts, we don't see any significant increase in CPU usage despite using coupled dynamics.
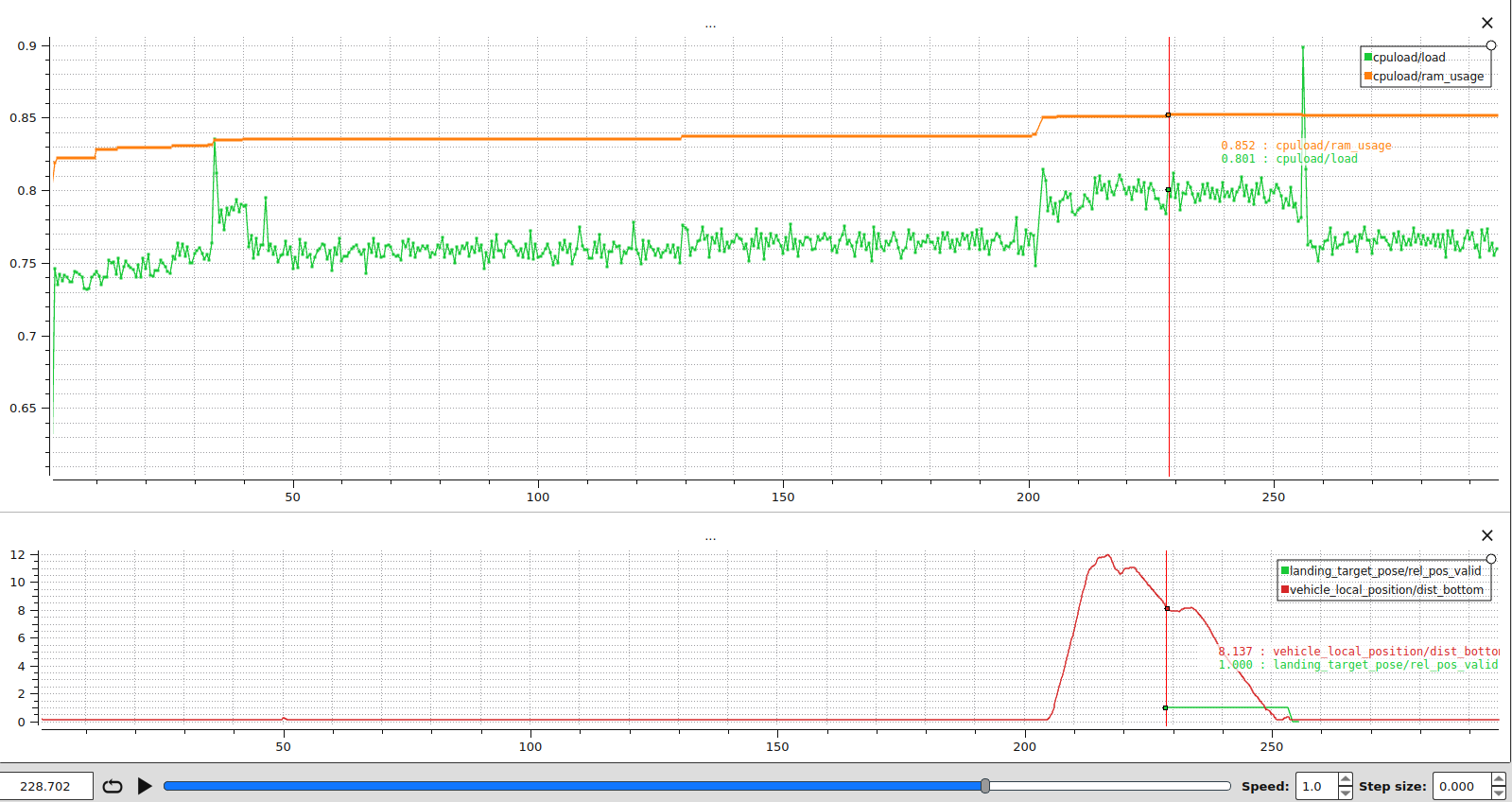
The innovations resemble white noise indicating good filter performances.
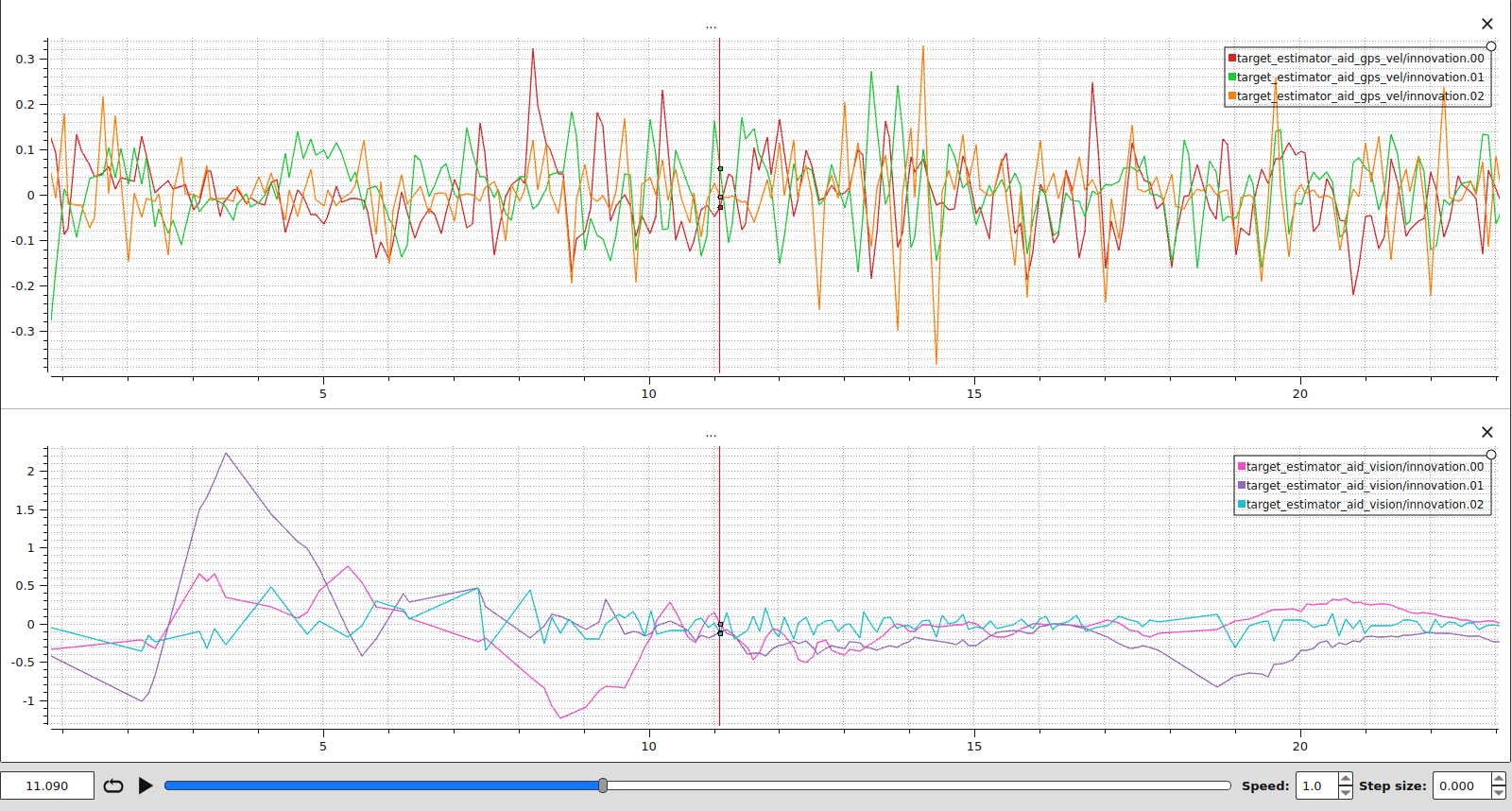
- Flight test with: stationary target, coupled dynamics fusing GPS velocity and external vision.
1. The target is moved while the drone is descending. Once the target has been moved, the vision measurements are correctly detected as outliers and thus not fused. At some point the filter times out ( `LTEST_BTOUT`) and restarts. As the target is still visible, the drone is able to correct and land on the target.
https://user-images.githubusercontent.com/74473718/203310158-797b214e-f753-4bc9-99b7-a5165c7151cf.mp4
2. Same behavior as in the previous video except that the landing target is not visible once the filter restarts. Therefore the drone falls back to normal landing
https://user-images.githubusercontent.com/74473718/203310214-4b99f259-052d-454c-9413-c1cf96993014.mp4
- Simulation stationary target, coupled dynamics fusing GPS vel and GPS mission position: [](https://logs.px4.io/plot_app?log=d48be859-db69-4f2a-98ea-6627fecb2405)
The drone takes off (origin of local frame) and lands at the same location, therefore we expect to see the local position of the drone and the local position of the target converge to zero.
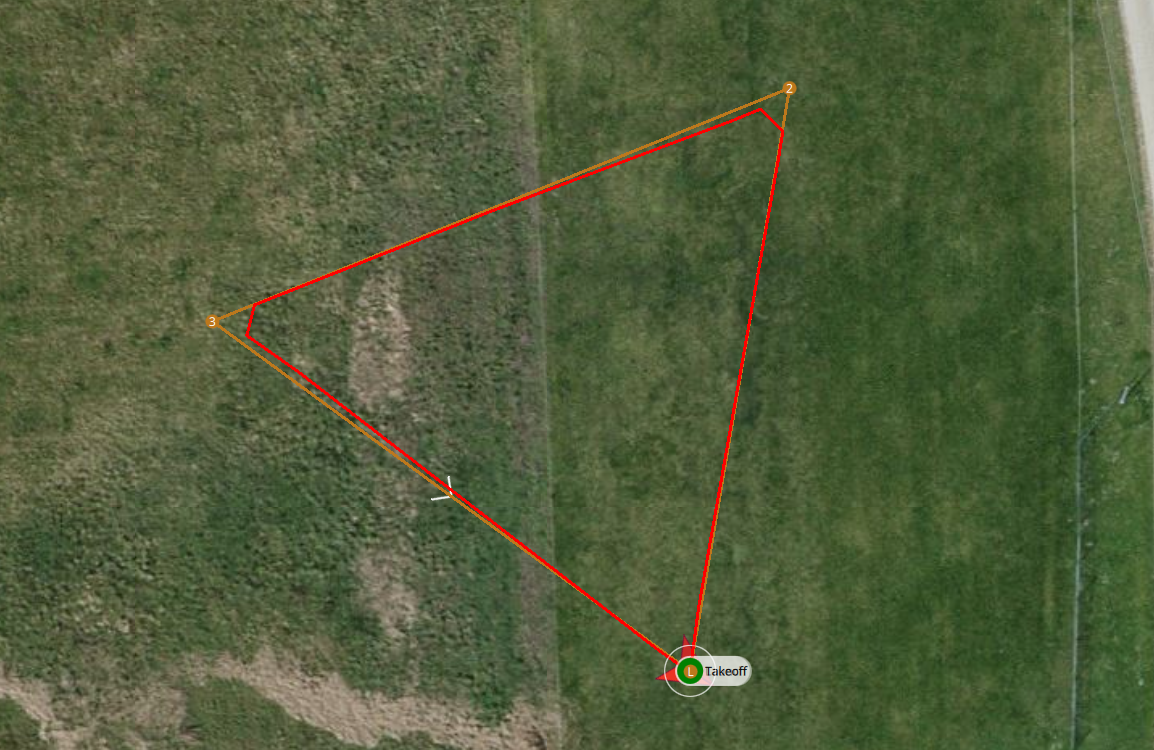
The relative position to the target is obtained using the drone's GPS position and the mission position. This plot shows that the relative position is properly computed.
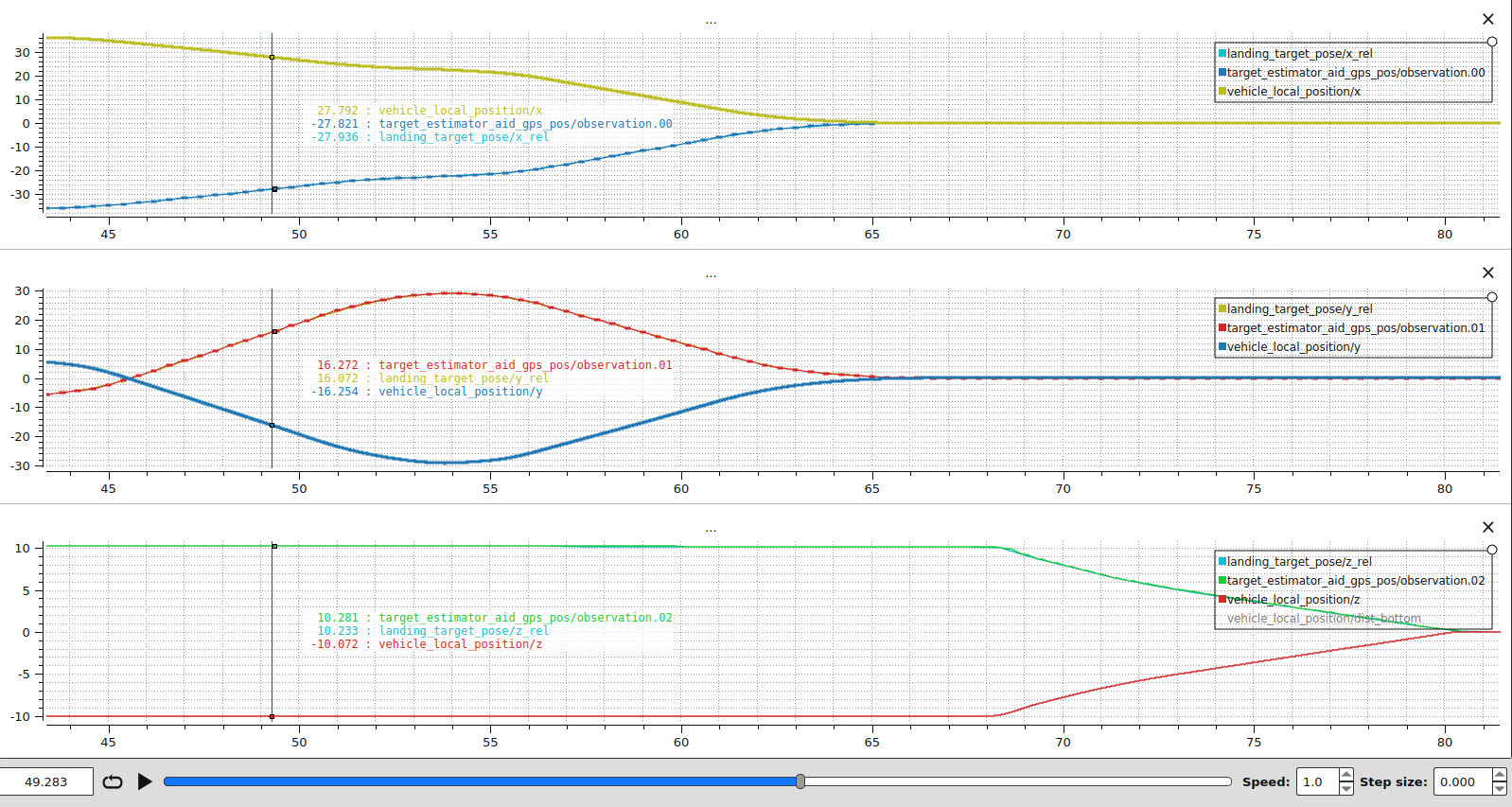
- Simulation stationary target, coupled dynamics fusing only GPS vel and using the GPS mission position to init the estimator:
The target estimator timeout is set to 10 seconds i.e. the filter will estimate the target position using only the initial position and the velocity measurement during 10 seconds. In the plots we cannot determine where the target estimator times out / restarts showing that the KF model works well.
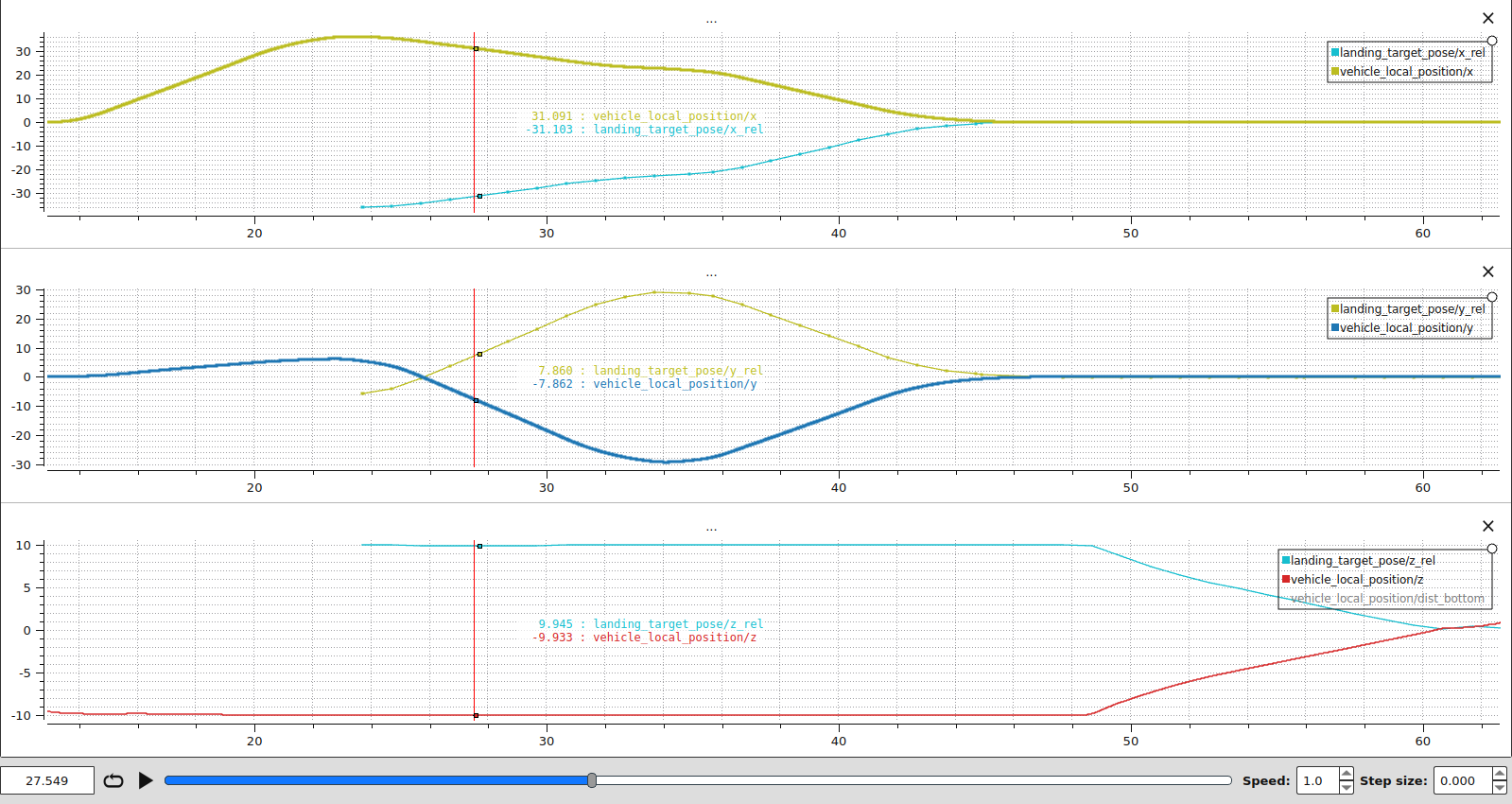
Note, if the gravity is not compensated, the prediction is not correct as shown in the bottom plot below.
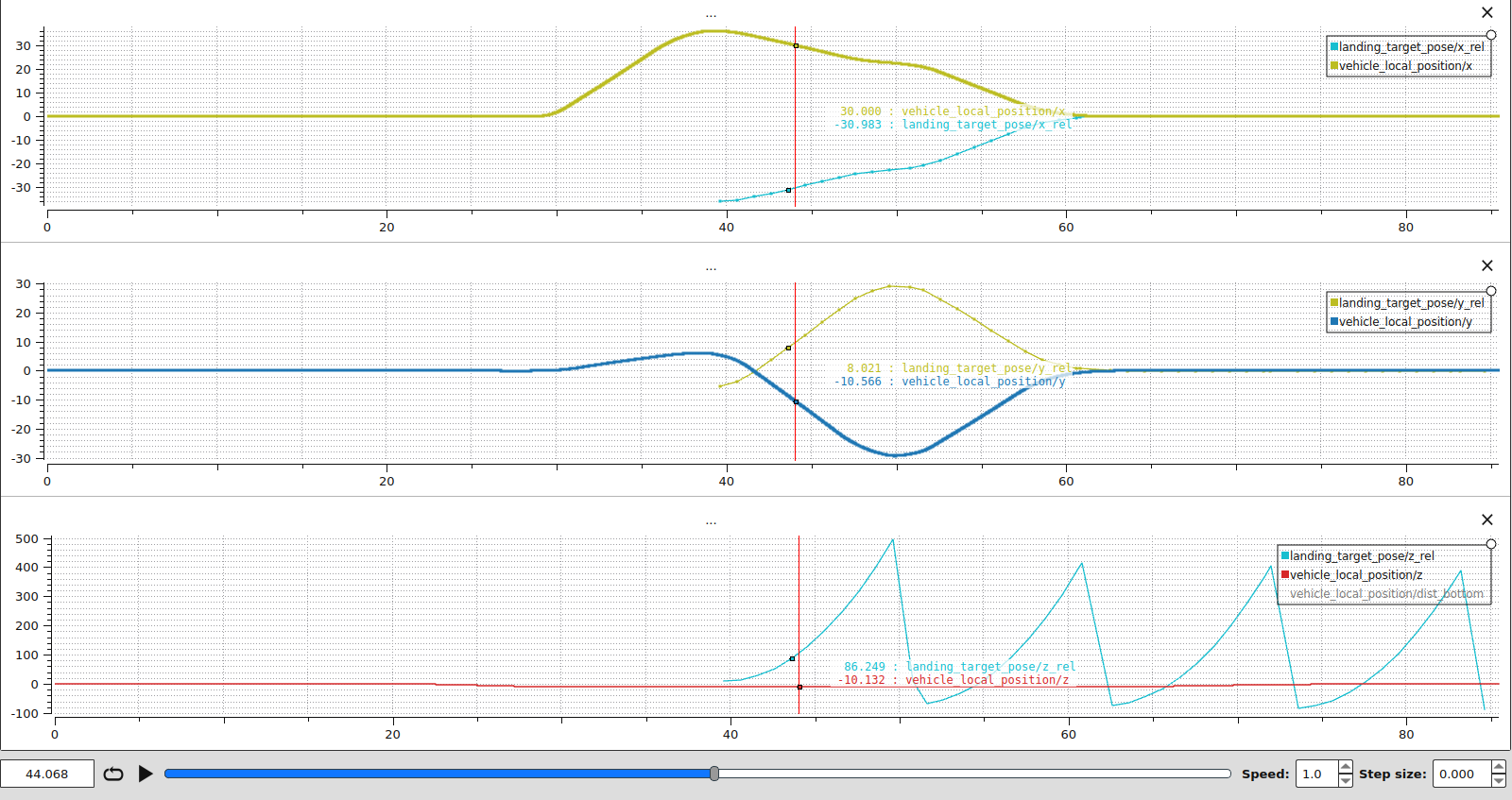
It might be worth correcting the current implementation https://github.com/PX4/PX4-Autopilot/tree/main/src/modules/landing_target_estimator by adding:
```
// Compensate for gravity:
Dcmf R_att_inv = inv(R_att);
Vector3f gravity_ned(0, 0, 9.807);
Vector3f gravity_body = R_att_inv * gravity_ned;
input->vehicle_acc_ned = R_att * (vehicle_acc + gravity_body);
```
2 Likes